The Revenue Blog /
Must-Know Sales Forecasting Methods to Predict Revenue
Must-Know Sales Forecasting Methods to Predict Revenue
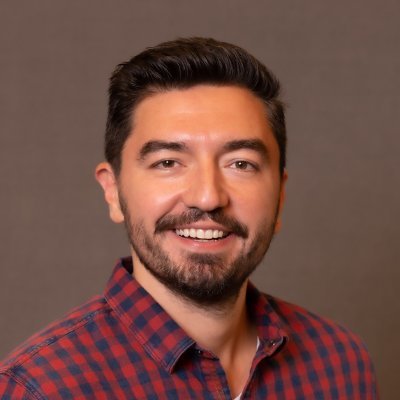
Batu Apaydin
December 20, 2023
7 min read
Topics covered in this article
Sales processes are in place, the sales staff are working on the deals. As a manager, you can track the status of opportunities in various reports.
But how do you turn this into a forecast?
What sales forecasting models are there to create accurate estimates for you and your peers?
In this article, we will explore this topic and illustrate the most important sales forecasting methods using examples.
What Are Sales Forecasting Models?
Sales forecasting techniques represent methodical approaches designed to accurately predict future sales.
These techniques enable businesses to anticipate market trends, facilitating more effective budget planning and operational strategizing. They provide a robust quantitative foundation for future sales predictions and the overall sales forecast strategy.
Prerequisites of Accurate Sales Predictions
- High-quality data from a diverse set of sources. Use data from CRM systems, account engagement data, like emails or calls, market research, and macroeconomic trends to get a comprehensive overview of customer behavior and sales patterns. This cross-source approach provides a richer and more accurate data set for forecasting.
- Minimize manually collected information. Rely on automated data collection and management to reduce human error and bias and increase the accuracy and efficiency of the data used for sales forecasting.
- Well-structured sales process. Implement a standardized process for sales activities to ensure uniform data collection at every stage. This consistency is key to building a reliable foundation for sales analysis.
- Well-defined KPIs for process monitoring. Establish clear Key Performance Indicators (KPIs) that track the effectiveness of each stage of the sales process, from lead generation to deal closure. Instead of relying only on KPIs related to the end result (eg. expected revenue), implement KPIs related to the complete sales cycle, including calls, emails, meetings, etc.
- Appropriately chosen methods of sales forecasting. Choose a forecasting method, whether quantitative or qualitative, that best fits the business's specific needs and data characteristics. The right method will accurately reflect business dynamics and market conditions and lead to more reliable forecasts.
Time Series Analysis as a Sales Forecasting Model
Time series analysis is one of the key sales forecast methods. It involves examining historical sales data to identify patterns and trends over time and using these insights to forecast future sales.
In this approach, historical data is usually divided into trend, seasonality, and noise. The trend indicates the long-term direction of the data, seasonality represents periodic fluctuations and noise includes random fluctuations.
The application of time series analysis in sales forecasting requires extensive and high-quality historical sales data. To apply this method effectively, data is required that captures trends, seasonal fluctuations, and other relevant sales influences.
Here are three exemplary scenarios in which time series analysis proves its worth:
-
Predicting retail sales. For a retail chain, analyzing past sales data helps predict seasonal fluctuations and trends to optimize inventory levels and marketing strategies.
-
E-commerce demand forecasting. Online stores can use time series analysis to predict sales peaks or slumps and adjust their inventory and pricing strategies accordingly.
-
B2B sales trend analysis. Companies can anticipate the buying behavior of corporate customers by analyzing past transaction data to support strategic planning and resource allocation.
In each scenario, the accuracy of the method depends on the quality and relevance of the historical data, which emphasizes its importance for strategic sales planning.
Random Forest and Gradient Boosted Decision Trees in Sales Forecast Modeling
Random Forest and Gradient Boosting are sophisticated machine-learning methods for predictive accuracy in sales forecast modeling.
Unlike linear regression models, which cannot adequately capture complex, non-linear relationships in the data, these methods are characterized by their ability to construct a series of decision trees to model different potential outcomes and trends.
A random forest creates a "forest" of decision trees, where each tree is created from a random subset of the data to ensure diversity. The predictions from each tree are then combined into a single, more accurate forecast. This method reduces the risk of overfitting, a common challenge where models work well on known data but cannot be generalized to new data.
Gradient boosting takes a different approach by starting with a single decision tree and gradually adding trees that correct the errors of the previous trees. Each new tree in the sequence focuses on the most difficult cases and improves the accuracy of the model with each step. This sequential correction results in a robust predictive model that can handle complex data sets with intricate patterns.
These techniques are particularly advantageous over linear models such as logistic regression when it comes to predicting business outcomes. They can uncover hidden patterns in the sales pipeline and activity that linear models may miss, leading to better decision-making. Examples of their application include:
- Strategic product placement. Tailoring inventory to consumer buying habits identified through pattern recognition.
- Market entry tactics. Assessing the potential success of new products or services in various markets.
- Advertising effectiveness. Evaluating the impact of marketing campaigns and adapting strategies accordingly.
By integrating Random Forests and Gradient Boosted Decision Trees into the analysis process, companies can significantly improve their forecasting models and ensure that they are well-equipped to respond to dynamic market demands and consumer behavior.
Logistic Regression for Sales Forecasting
Logistic regression, a more transparent analysis tool in the sales forecasting strategy, is used to determine the probability of sales outcomes. It's an approach that estimates the probability of a particular event, such as a sale, based on given predictors. The data required for this method includes historical sales figures, customer demographics, marketing spending, and sales team activity.
Practical applications of logistic regression in sales forecasting include:
- Market segmentation analysis. Predicting customer segments that are likely to make a purchase.
- Effectiveness of promotions and campaigns. Estimating the success rate of various sales promotions.
- Sales funnel conversion. Assessing the likelihood of leads converting to sales at different stages of the sales funnel.
This method provides a quantifiable way of measuring the impact of various factors on sales success and helps with strategic decision-making.
Conclusion
When selecting the ideal sales forecast model, it is important to harmonize the capabilities of the method with the company-specific data and objectives.
Time series analysis is suitable for companies with extensive historical data, while random Forest and Gradient Boosting are geared toward complex patterns and relationships. The right choice balances data availability, desired forecast granularity, and the nature of sales cycles, ensuring customization to the company's unique sales ecosystem.